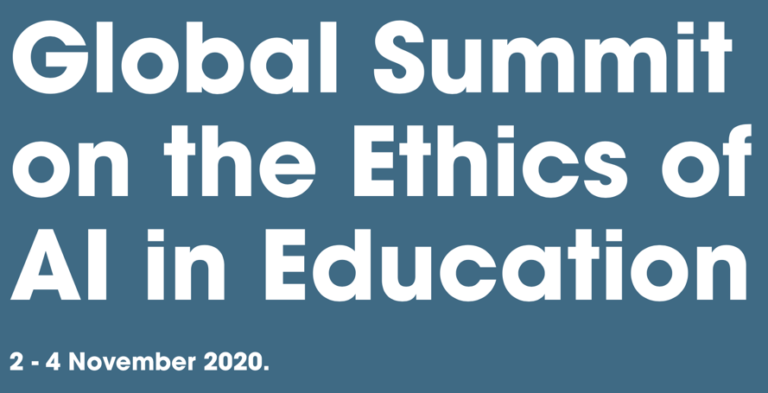
During the first week of November 2020, I participated in the Global Summit on the Ethics of AI in Education. Leaders in the field of artificial intelligence in education (AIED) got together virtually to discuss key ethical questions regarding the use of AI in education. I took part in discussions on how to facilitate the ethical use of AI in education, drawing on our experience and approach at Alelo.
AI-driven learning products are expanding their role in education. Teachers, parents, and other stakeholders want to be sure that they can trust AI to help students and not introduce risks and negative side effects. The failure to consider the potential negative effects of AI in the classroom has sometimes led to a backlash from parents, students, and teachers.
Unfortunately, there is not yet a single agreed-upon set of ethical principles that AIED developers can follow. A variety of professional organizations and private companies have each published their own sets of principles. A recent analysis of ethical AI principles published in the Harvard Data Science Review focused on six high-profile initiatives established to promote socially beneficial AI, which together articulated 47 ethical principles. The analysis converged on five core principles, each of which is relevant to AI in education and Alelo products in particular.
Core Principle 1: Beneficence
AI technology should be used in a manner that is beneficial to humanity. This would appear to be uncontroversial, yet well-intentioned software developers can still unintentionally create AIED systems with harmful side effects. To guard against this, Alelo’s avatar technology adheres to all core ethical principles identified in the Harvard Data Science Review, including those listed below.
Core Principle 2: Non-Maleficence
AI technology must not just do good, but also do no harm. Violation of privacy is one kind of harm, and to avoid this, Alelo scrupulously follows international standards in data protection. Personalized learning software that optimizes learning for students potentially harms students if it excludes teachers from the instructional process. We design our Enskill® platform to be used in a blended fashion by both students and teachers, so that students benefit from teacher guidance as well as instructional algorithms.
Core Principle 3: Autonomy
AI should respect the autonomy of users to make their own decisions. For example, Enskill gives students recommendations of what exercises to work on but lets the students and teachers make the final decision. Teachers have access to student performance analytics as well as the underlying learner data, so they can make their own judgments about student learning. Alelo also provides professional development resources to teachers so that they understand how to make the best use of avatar technology.
Core Principle 4: Justice
AIED systems should support students in a fair and equitable manner. This principle informs the design of Enskill as follows. We train Enskill AI models on data from a variety of students around the world, to avoid biasing the models toward specific categories of students. We offer low-bandwidth solutions and solutions on a variety of devices, to benefit students with limited Internet access.
Core Principle 5: Explicability
Because teachers have access to Enskill’s student data and analytics, Enskill’s instructional decisions are transparent to teachers. We avoid using black-box AI algorithms where it might adversely affect teachers’ trust in the technology.
By adhering to these principles, Alelo avatar-based learning systems support a wide range of learners, and avoid the possible negative side effects that can arise when AI is applied inappropriately.
About The Author
Lewis Johnson
Dr. W. Lewis Johnson is President of Alelo and an internationally recognized expert in AI in education. He won DARPA’s Significant Technical Achievement Award and the I/ITSEC Serious Games Challenge, and was a finalist in XPRIZE Rapid Reskilling. He has been a past President of the International AI in Education Society, and was co-winner of the 2017 Autonomous Agents Influential Paper Award for his work in the field of pedagogical agents. He is regularly invited to speak at international conferences for distinguished organizations such as the National Science Foundation.